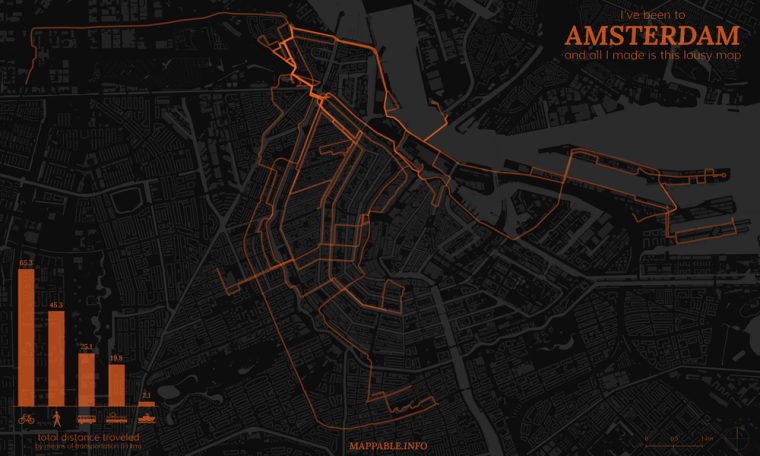
As you’ve probably read here before, I (Patrick) am currently on a cartography / dataviz journey around the world. I originally planed to do a lot of mapping during the trip, but didn’t have enough time to do so recently. Too many interesting places to visit and too many short stops so far (which will change soon).
But just after spending 9 days in Amsterdam at the beginning of August, I used the bus ride to Hamburg, and a few more hours later on, for making a map of all my tracks during the stay in Amsterdam. As I didn’t have any special equipment and barely any time to get prepared, this had to work with a very simple approach. If you’re interested in a more sophisticated way of tracking your travels, take a look at Bjørn Sandvik’s blog thematicmapping.
I simply tracked my movement with a smartphone GPS and the OpenPaths service. While I had good experiences with this setup in Germany, it didn’t quite work out abroad. At that time I travelled without a data plan, which meant that my phone couldn’t make use of the A-GPS (Assisted GPS) function, which significantly improves GPS performance. What did that mean for me? Well, a lot of my position data was just useless, the GPS deviations were way too high. But I didn’t want to be stopped by that. Amsterdam and it’s channels (dutch: ‘Grachten’) just look too beautiful on a map to skip this one.
What I ended up doing, was taking the GPS positions as a starting point, made lines out of continuos points and correcting/completing them in QGIS. This took me about two hours, and while the result is not 100% precise, it’s fair enough for a few hours of work and for a first try. The next map of this kind on one of my future stops will be better and the data collection method will improve as well, I promise.
Having complete coverage of my tracks, I categorized the lines according to the means of transportation I’ve used, measured their lengths and added it up to get my personal modal split for my stay in Amsterdam. As you would expect from a city with great bicycle infrastructure, and perfect weather at that time, I ended up using the bike most of the time. For finishing my map, I left QGIS and added the chart and the title in Adobe Illustrator. You can see the final result below, or here in a high-res, zoomable view. And yes, it’s the Netherlands, so it had to be orange/oranje!
Not to forget: the individually designed basemap, with it’s buildings and channels, is based on OpenStreetMap data (© OpenStreetMap contributors), which I’ve extracted via the Overpass API and the XAPI Query Builder. OSM data is available under the Open Database License.